The stories about how artificial intelligence can improve our lives and how to make the company progress will surprise us every day. Artificial intelligence has analyzed X-rays, powered the Internet of Things, and recommended the next best action plan for sales and marketing teams. It seems that there are still infinite possibilities.
But behind every successful story of artificial intelligence, countless projects have died in the lab. This is because putting machine learning research into production and using it to provide real value to customers is often more difficult than developing a scientifically sound algorithm. In the past few years, many of the companies I have encountered have faced this challenge, which I call "across the artificial intelligence divide."
In this article, I will share my four lessons in overcoming my technical and product difficulties from your perspective. I recently proposed these points on ApacheCon.
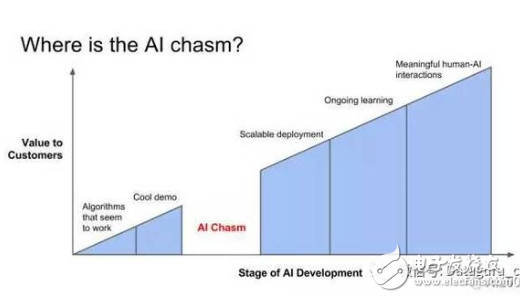
Artificial intelligence technology gap
New data. Data is the key to artificial intelligence. For example, if you want a chat bot to learn, you must provide sample data of the client's request and the corresponding correct response to its algorithm model. Samples are usually static, structured data, such as the CSV data format.
While you can build cool artificial intelligence presentations using static datasets, real-world artificial intelligence algorithm models require new data for incremental training to make it smarter over time. That's why companies should invest in machine learning architectures early, collect new data constantly, and use it to regularly update their artificial intelligence models.
The use of real-time data faces many engineering challenges, including scheduling, non-stop model iteration, stability and performance monitoring. Also, if there is a problem with the new data, you need a mechanism to go back to the previous state and take us to the next point.
Ensure the quality of the training data collected. Companies should consider the quality of data from the start - especially from user-generated data. Machine learning automation is an exciting thing, but it can also be counterproductive. Recently, some chat bots on Twitter have become very rogue, which is an example of typical automation variation.
Before the chatbot begins to talk freely, it trains with publicly modeled, cleaned, and filtered data. But after the robot began to learn from inappropriate communication with real people, the tone of its tweet quickly became worse. GIGO (garbage in, garbage out) is the basic rule of machine learning, so a good artificial intelligence system can detect potential problems and alert administrators when manual intervention is required.
Artificial intelligence product divide
Optimize the right goals. The success of artificial intelligence depends on correctly defining your prediction problems. From the beginning, you need to clearly identify the input query, output the prediction, and distinguish which predictions are good and which predictions are bad. Data scientists will use these assessment indicators to determine the accuracy of the artificial intelligence model.
First set your goals. Do you want to maximize revenue, create a better user experience, automate manual tasks or other tasks? To be successful, real-world artificial intelligence products must use metrics that accurately reflect business goals.
Netflix's story of the recommended algorithmic competition gave everyone a lesson. After the creator of a new movie rating algorithm was awarded a $1 million prize, Netflix was unable to use the algorithm on a large scale in the real world, mainly because the transition from DVD to video stream made the original target meaningless.
When setting up metrics, keep in mind three key requirements. You need to ensure that: (1) measure what is really important; (2) use real-time data and new data to evaluate results; and (3) interpret the results to stakeholders in a way that they understand and value. The last requirement points to the key points of human and artificial intelligence interaction.
Humans interact with artificial intelligence. Humans are very complicated. Therefore, when they interact with artificial intelligence, they encounter new challenges that are not encountered when processing data sets in the lab. Please note that if the customer does not trust artificial intelligence, then the product it drives will not be used. Although you can try to build trust by showing the accuracy of the predictive model, most consumers can't really focus on reliable scientific indicators.
Therefore, you need to use the user experience/user interface (UX / UI) of your product to overcome the difficulty of building trust. For example, when Apple's Siri Virtual Assistant first launches, it defaults to male or female voice depending on the country and region in which the user is located. Google’s self-driving models appear with a cute and friendly face to appease customers who are worried about their safety. Remember, people using your algorithm will not only bring you challenges, but also provide you with a solution.
In fact, the gap across artificial intelligence is not so frightening. As you move into this chasm, make sure you have a good plan to cross it instead of falling into the chasm. And remember that to become a pioneer in artificial intelligence, your company must adhere to the principle of customer first.
The author, Simon Chan, is a former co-founder/CEO of PredicTIonIO and currently serves as senior director of Salesforce Einstein's product operations.
Food Packaging Staple
Food Packaging Staple,Household Office Staples,Dainty Durable Staple,Narmal Packing Nails
Zhejiang Best Nail Industrial Co., Ltd. , https://www.beststaple.com