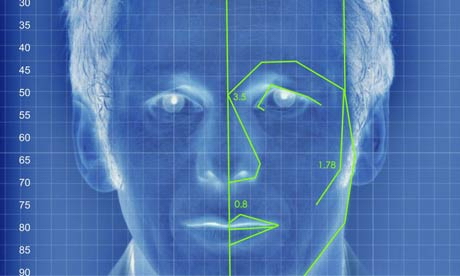
Face recognition refers specifically to computer technology that uses identity analysis to compare facial features. Face recognition is a popular field of computer technology research. It belongs to biometric identification technology. It is the biological characteristics of organisms (generally specific to humans) to distinguish organisms.
The generalized face recognition actually includes a series of related technologies for constructing a face recognition system, including face image acquisition, face location, face recognition preprocessing, identity verification, and identity search; and narrow face recognition specifically refers to Face recognition technology or identity search technology or system.
Biological characteristics studied by biometrics include human faces, fingerprints, palm prints, palms, irises, retinas, veins, voice (speech), body shape, infrared thermograms, ear patterns, odors, and personal habits (such as keystrokes Strength and frequency, signature, gait, etc., the corresponding recognition techniques are face recognition, fingerprint recognition, palmprint recognition, iris recognition, retina recognition, vein recognition, speech recognition (using voice recognition to identify, can also be For the recognition of voice content, only the former belongs to biometrics technology, body shape recognition, keystroke recognition, signature recognition, etc.
Geometric features of face recognition methods Geometric features can be the shape of the eyes, nose, mouth, etc., and the geometric relationships between them (such as the distance between each other). These algorithms are fast and require little memory, but their recognition rate is low.
Face Recognition Method Based on Feature Face (PCA) The feature face method is a face recognition method based on KL transform, and KL transform is an optimal orthogonal transform of image compression. After the KL transformation, the high-dimensional image space obtains a set of new orthogonal bases, and retains the important orthogonal bases. These bases can be transformed into low-dimensional linear spaces. If it is assumed that the projections of human faces in these low-dimensional linear spaces are separable, these projections can be used as the identified feature vectors. This is the basic idea of ​​the eigenface method. These methods require more training samples and are entirely based on the statistical properties of the grayscale of the image. There are currently some improved feature face methods.
The face recognition method of the neural network The input of the neural network may be a face image with reduced resolution, an autocorrelation function of a local region, a second moment of local texture, and the like. This kind of method also needs more samples to train, but in many applications, the number of samples is very limited.
The elastic map matching face recognition method elastic map matching method defines a distance in the two-dimensional space that has certain invariance for the normal face deformation, and uses the attribute topological map to represent the face, the topology map Each vertex contains an eigenvector used to record information about the face near the vertex position. The method combines grayscale features and geometric factors, allows the image to be elastically deformed when compared, and achieves good results in overcoming the effects of expression changes on recognition. At the same time, it does not require multiple samples for a single person. training.
Psychology studies of line segment Hausdorff distance (LHD) face recognition methods show that humans are no worse than recognizing grayscale images in identifying the speed and accuracy of contour maps (such as comics). LHD is based on the line segment diagram extracted from the face grayscale image. It defines the distance between two line segment sets. What is different is that LHD does not establish a one-to-one correspondence between line segments of different line segment sets. Relationships, so it is more adaptable to small changes between line segments. The experimental results show that LHD has excellent performance under different lighting conditions and different postures, but it does not perform well in large-expression situations.
Face Recognition Method Based on Support Vector Machine (SVM) In recent years, support vector machine (SVM) has become a new hot spot in the field of statistical pattern recognition. It tries to make the learning machine compromise on experience risk and generalization ability, thereby improving the learning machine. Performance. Support vector machine (SVM) mainly solves a 2-category problem. Its basic idea is to try to transform a low-dimension linearly inseparable problem into a high-dimensional linearly separable problem. The usual experimental results show that SVM has a good recognition rate, but it requires a large number of training samples (300 per class), which is often unrealistic in practical applications. Moreover, the support vector machine has a long training time, the method is complicated to implement, and there is no unified theory for the retrieval of the kernel function.
Face recognition technology The traditional face recognition technology is based on visible light image face recognition. This is the most familiar recognition method. It has more than 30 years of research and development history. However, this method has insurmountable deficiencies, especially when the ambient light changes, the recognition effect will drop sharply, and it cannot meet the needs of the actual system. Solutions to solve the lighting problem include three-dimensional image face recognition and thermal imaging face recognition. However, these two technologies are still far from mature, and the recognition effect is not satisfactory.
One solution that has recently been rapidly developed is a multi-light source face recognition technology based on active near-infrared images. It can overcome the effects of light changes and has achieved excellent recognition performance. The overall system performance in terms of accuracy, stability, and speed exceeds 3D image face recognition. This technology has developed rapidly in the past two or three years, making the face recognition technology gradually practical.
Digital camera face autofocus and smile shutter technology is the first face capture. Based on the position of the person's head, the head is first determined, and then the head and mouth features are judged. Through the comparison of the feature database, it is confirmed that the face is human face and the facial capture is completed. Then focus on the human face to focus, you can greatly improve the clarity of the photos taken. Smiley shutter technology is based on face recognition, complete facial capture, and then began to determine the degree of curvature of the mouth and the degree of eye bending, to determine whether it is a laugh. All of the above captures and comparisons are done in the context of contrasting feature libraries, so the feature library is the foundation, with a variety of typical face and smile feature data.
Jiangsu Jiangyang Special Cable Co,.Ltd. , https://www.jymarinecable.com